Fine-tuning models have gained momentum with the advent of transfer learning, particularly in natural language processing (NLP). Instead of starting from scratch, practitioners tweak sophisticated models initially developed by tech giants like Google or Microsoft.
These models, trained on vast datasets, provide an excellent starting point. Think of it as adjusting, rearranging, or swapping some LEGO pieces to better fit specific needs or to create something a bit unique.
In this blog post, you will explore the details of model fine-tuning, covering real-life scenarios, techniques, challenges, metrics, and best practices.
Fundamentals of ML Model Fine-tuning
Model fine-tuning involves refining pre-trained models for specific tasks. Unlike model training, which builds from scratch, fine-tuning adjusts existing models, saving time and resources.
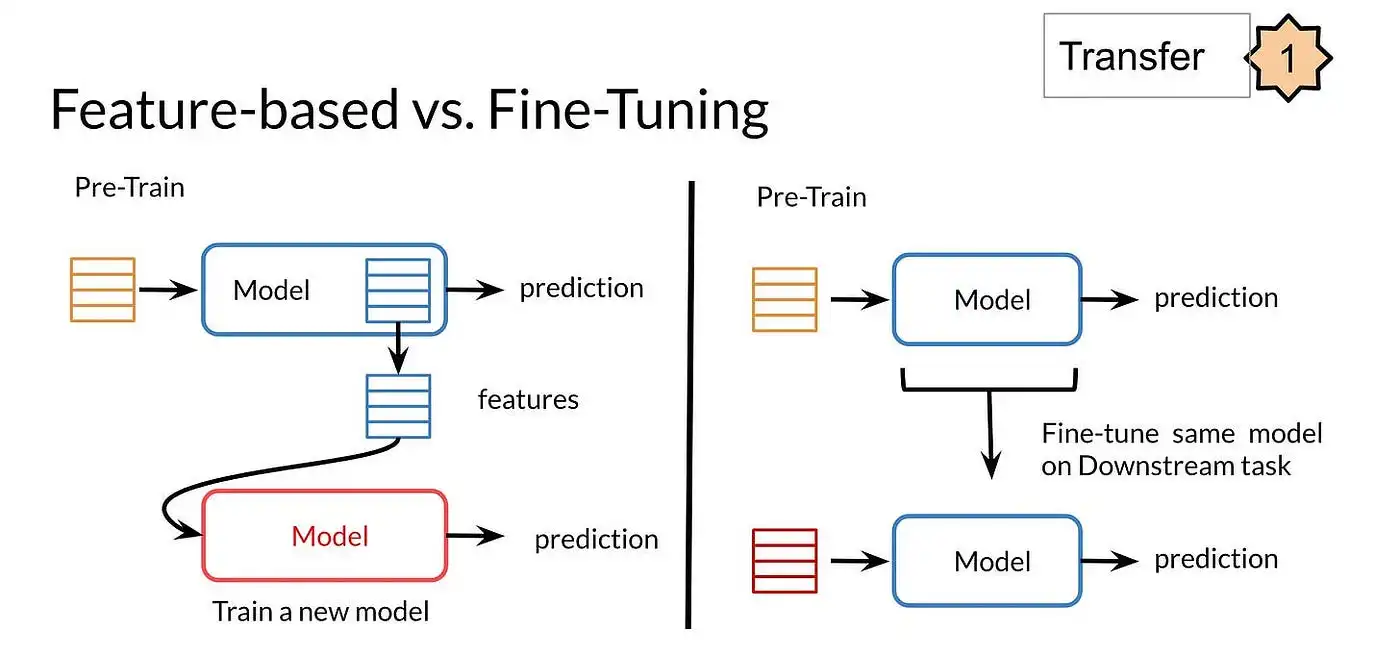
Practitioners might face challenges like overfitting and finding the right balance in adjustments. Key techniques of fine-tuning include hyperparameter tuning and regularization, aimed at optimizing performance.
Metrics are crucial for measuring improvements. Adhering to best practices, such as careful selection of training data and incremental adjustments, ensures effective fine-tuning. This process is integral for achieving higher accuracy and efficiency in ML models, leveraging the groundwork laid by extensive pre-training.
Model Fine-Tuning Vs. Model Training
The core distinction between model training and fine-tuning lies in their approach to developing machine learning models. While training builds a model from ground zero, fine-tuning tweaks an existing, pre-trained model for specific needs.
Here’s a practical comparison of Model Fine-tuning vs Model training:
Aspect | Model Training | Model Fine-tuning |
---|---|---|
Starting Point | Begins with a blank slate, no prior knowledge | Starts with a pre-trained model |
Data Requirements | Requires large, diverse datasets | Can work with smaller, specific datasets |
Time and Resources | Often time-consuming and resource-intensive | More efficient, leverages existing resources |
Objective | To create a general model capable of learning from data | To adapt a model to perform better on specific tasks |
Techniques | Involves basic learning algorithms, building layers, setting initial hyperparameters | Involves hyperparameter tuning, regularization, adjusting layers |
Challenges | Needs extensive data to avoid overfitting, underfitting | Risk of overfitting to new data, maintaining balance in adjustments |
Metrics | Focuses on overall accuracy, loss metrics | Emphasizes improvement in task-specific performance |
Best Practices | Requires careful data preprocessing, model selection | Necessitates cautious adjustments, validation of new data |
Techniques for Model Fine-tuning
Each of the following techniques addresses specific challenges in model fine-tuning, contributing to the creation of more accurate and reliable machine-learning models.
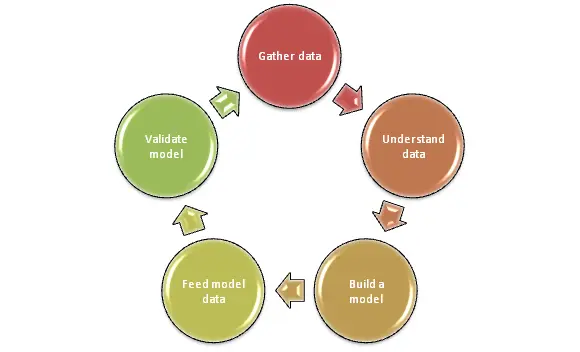
They are often used in combination to achieve the best results, tailored to the specific requirements of the task at hand.
1. Hyperparameter Tuning
This involves adjusting the model’s parameters to improve performance. For example, in a neural network, tuning the learning rate or batch size can significantly impact accuracy.
A practical case uses a grid or random search to find the optimal hyperparameters for a classification task.
2. Transfer Learning
Leveraging pre-trained models and adapting them to new tasks is a common fine-tuning method. For instance, using a model trained on a large image dataset to improve performance on a smaller, specialized image classification task.
3. Data Augmentation
Enhancing the training dataset by creating modified versions of data points helps in reducing overfitting. In image processing, this might mean rotating, flipping, or adding noise to images to create a more robust model.
4. Regularization Methods
The techniques, like L1 or L2 regularization, prevent overfitting by penalizing complex models. Adding a regularization term to the loss function can maintain model simplicity and improve generalization.
Overcoming Challenges of fine-tuning ML Models
ML Model Fine-Tuning Challenges
Fine-tuning ML models comes with its unique set of challenges, which practitioners must adeptly navigate:
- Risk of Overfitting: Fine-tuning often risks making the model too specific to the new data, losing its generalization ability. To counter this, implementing cross-validation and using techniques like regularization can help maintain the model’s ability to perform well on unseen data.
- Resource Constraints: Fine-tuning, especially on large models, demands significant computational resources. Effective strategies include using cloud-based computing solutions or optimizing model architecture to reduce resource demands.
- Hyperparameter Complexity: Choosing the right hyperparameters is crucial yet challenging. Utilizing automated hyperparameter optimization techniques, such as Bayesian optimization, can simplify this process.
Ways to Overcome the Challenges
By adopting the following best practices and leveraging the right techniques, practitioners can fine-tune models efficiently while minimizing risks and resource overhead.
- Cross-Validation: This technique involves dividing the dataset into multiple parts to validate the model’s performance on different subsets. It helps in assessing the model’s generalization ability, reducing the risk of overfitting.
- Regularization: Techniques like L1 and L2 regularization penalize model complexity, thereby preventing overfitting. They work by adding a penalty to the loss function based on the magnitude of the model parameters.
- Efficient Hyperparameter Optimization: Approaches like grid search, random search, or more advanced methods like Bayesian optimization can effectively find optimal hyperparameters without extensive manual tuning, making the fine-tuning process more efficient and less resource-intensive.
ML Model Fine-tuning Metrics
Evaluating the effectiveness of optimized machine learning models is crucial for understanding their performance. Various metrics are commonly employed to quantify the success of fine-tuned models. Let’s delve into some key metrics along with examples to illustrate their significance.
1. Accuracy
This metric measures the proportion of correct predictions among the total number of cases evaluated. For instance, in a disease diagnosis model, if 90 out of 100 diagnoses are correct, the accuracy is 90
2. Precision
Precision assesses the correctness of positive predictions. In a spam detection model, if out of 100 emails marked as spam, 80 are spam, the precision is 80
3. Recall
This metric calculates how many actual positives were correctly identified. Using the same spam model, if 100 spam emails exist and the model correctly identifies 90, the recall is 90
4. F1 Score
F1 Score combines precision and recall into a single metric. It’s particularly useful in imbalanced datasets. For example, in fraud detection, where frauds are rare, a high F1 score ensures a high recall of fraud cases and precision in correctly identifying them.
5. Mean Absolute Error (MAE)
MAE calculates the average size of mistakes in predictions, disregarding whether they are over or underestimated. For example, if the true values are [3, -0.5, 2, 7] and predictions are [2.5, 0.0, 2, 8], the MAE is 0.5, indicating a small average error across predictions.
6. Root Mean Squared Error (RMSE)
RMSE is a quadratic scoring rule that also measures the average magnitude of the error. It gives a relatively high weight to large errors. If the RMSE of a temperature forecast model is 5 degrees, it indicates a higher penalty for large errors compared to MAE.
Best Practices
Adhering to best practices in model fine-tuning ensures the development of robust and effective machine-learning models:
- Transfer Learning: Utilize pre-trained models as a starting point to save time and resources, particularly beneficial for tasks with limited data.
- Data Augmentation: Expand training datasets by introducing variations, enhancing the model’s ability to generalize, and reducing overfitting.
- Regularization: Implement techniques like L1 and L2 regularization to prevent overfitting by penalizing model complexity.
- Grid Search and Random Search: Employ these methods for systematic hyperparameter optimization, balancing thoroughness (grid search) and randomness (random search).
- Ensemble Methods: Combine multiple models to improve predictions, leveraging the strengths of diverse approaches.
- Model Interpretability: Focus on making models understandable, aiding in troubleshooting and trust-building among stakeholders.
- Documentation: Maintain comprehensive documentation of model development processes for transparency and future reference.
- Collaboration: Foster collaboration between team members, benefiting from diverse perspectives and expertise in problem-solving and innovation.
Final Thoughts
In conclusion, fine-tuning ML models emerges as a nuanced and highly effective strategy for elevating the performance of machine learning tasks. Techniques such as hyperparameter tuning, data augmentation, and regularization play pivotal roles in tailoring these models to specific tasks, fostering improved model accuracy.
Markov, provides powerful Experiment and Evaluation features, enabling you to systematically monitor and assess your model’s performance on your selected dataset. This functionality empowers you to keep a detailed record of experiments and evaluations, facilitating iterative refinement for enhanced precision and accuracy.
Through the Markov dashboard, you gain access to intuitive graphical representations of all conducted experiments and evaluations. This visual overview simplifies the analysis process, making it effortless to identify trends and patterns. This visual insight is invaluable for making informed decisions on fine-tuning your model to achieve optimal results.